
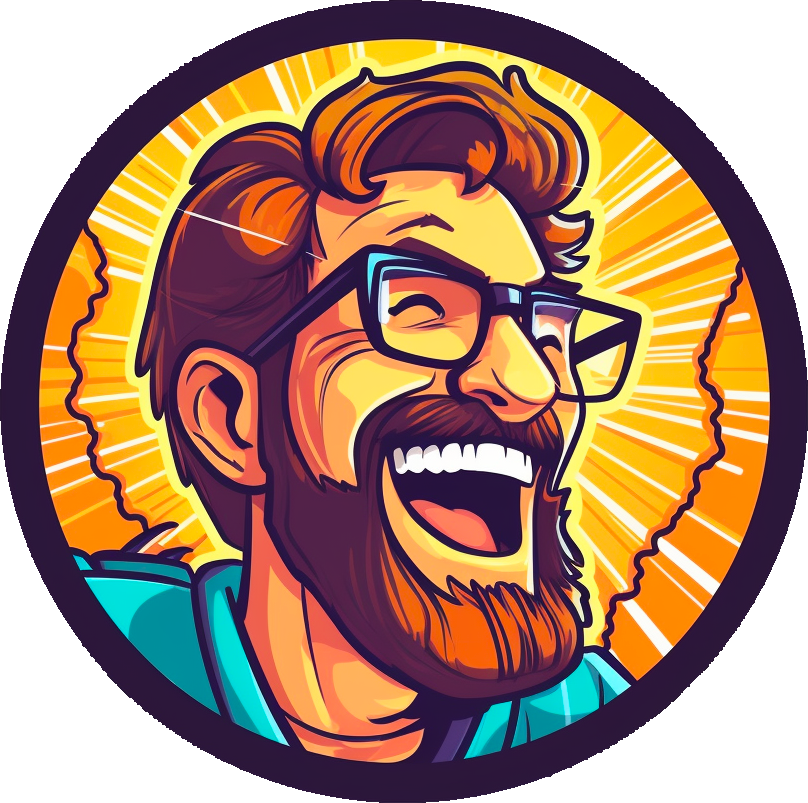
Or a few more gb of LLM?
Or a few more gb of LLM?
No, all sizes of llama 3.1 should be able to handle the same size context. The difference would be in the “smarts” of the model. Bigger models are better at reading between the lines and higher level understanding and reasoning.
Wow, that’s an old model. Great that it works for you, but have you tried some more modern ones? They’re generally considered a lot more capable at the same size
Increase context length, probably enable flash attention in ollama too. Llama3.1 support up to 128k context length, for example. That’s in tokens and a token is on average a bit under 4 letters.
Note that higher context length requires more ram and it’s slower, so you ideally want to find a sweet spot for your use and hardware. Flash attention makes this more efficient
Oh, and the model needs to have been trained at larger contexts, otherwise it tends to handle it poorly. So you should check what max length the model you want to use was trained to handle
I still use http a lot for internal stuff running in my own network. There’s no spying there… I hope … And ssl for local network only services is a total pita.
So I really hope browsers won’t adapt https only
But even if you use GoMommy extra super duper triple snake oil security checked ssl cert, if I trick LetsEncrypt to sign a key for that domain I still have a valid cert for your site.
I doubt the disk will bottleneck at 40mb/s when doing sequential write. Torrent downloads are usually heavy random writes, which is the worst you can do to a HDD.
I was trying to find an article I read about a year ago, about an experiment where AI was assisting a doctor. Where it suggested questions and possible diagnosis for the doctor to look into.
IIRC the result was both faster and more accurate diagnosis. Too bad I can’t find it again now :(
You’re not great taking medical advice from a doctor either, seeing how often they’re wrong.
I remember back in the day this automated downloader program… the links had a limit of one download at a time and you had to solve a captcha to start each download.
So the downloader had built in “solve other’s captcha” system, where you could build up credit.
So when you had say 20 links to download you spent some minutes solving other’s captchas and get some credit, then the program would use that crowdsourcing to solve yours as they popped up.
Llama3 8b can be run at 6gb vram, and it’s fairly competent. Gemma has a 9b I think, which would also be worth looking into.
And just to top it off, make this pythonscript a dialect of rust
Better background backups
Rework background backups to be more reliable
Hilarious for a system which main point / feature is photo backup
🫰🤙🫵👌✊🫳🫸🤲🤌
I worked on one where the columns were datanasename_tablename_column
They said it makes things “less confusing”
I mean, I totally agree with you. But that also kinda ignores all the useful things a dog can be trained to do.
It’s less the calculations and more about memory bandwidth. To generate a token you need to go through all the model data, and that’s usually many many gigabytes. So the time it takes to read through in memory is usually longer than the compute time. GPUs have gb’s of RAM that’s many times faster than the CPU’s ram, which is the main reason it’s faster for llm’s.
Most tpu’s don’t have much ram, and especially cheap ones.
Reasonable smart… that works preferably be a 70b model, but maybe phi3-14b or llama3 8b could work. They’re rather impressive for their size.
For just the model, if one of the small ones work, you probably need 6+ gb VRAM. If 70b you need roughly 40gb.
And then for the context. Most models are optimized for around 4k to 8k tokens. One word is roughly 3-4 tokens. The VRAM needed for the context varies a bit, but is not trivial. For 4k I’d say right half a gig to a gig of VRAM.
As you go higher context size the VRAM requirement for that start to eclipse the model VRAM cost, and you will need specialized models to handle that big context without going off the rails.
So no, you’re not loading all the notes directly, and you won’t have a smart model.
For your hardware and use case… try phi3-mini with a RAG system as a start.
So you’re saying it’s already feature complete with most json libraries out there?
And then it doesn’t work on one VM and it takes you three days to figure out that VM had a slightly different version of a library and that makes the app segfault.